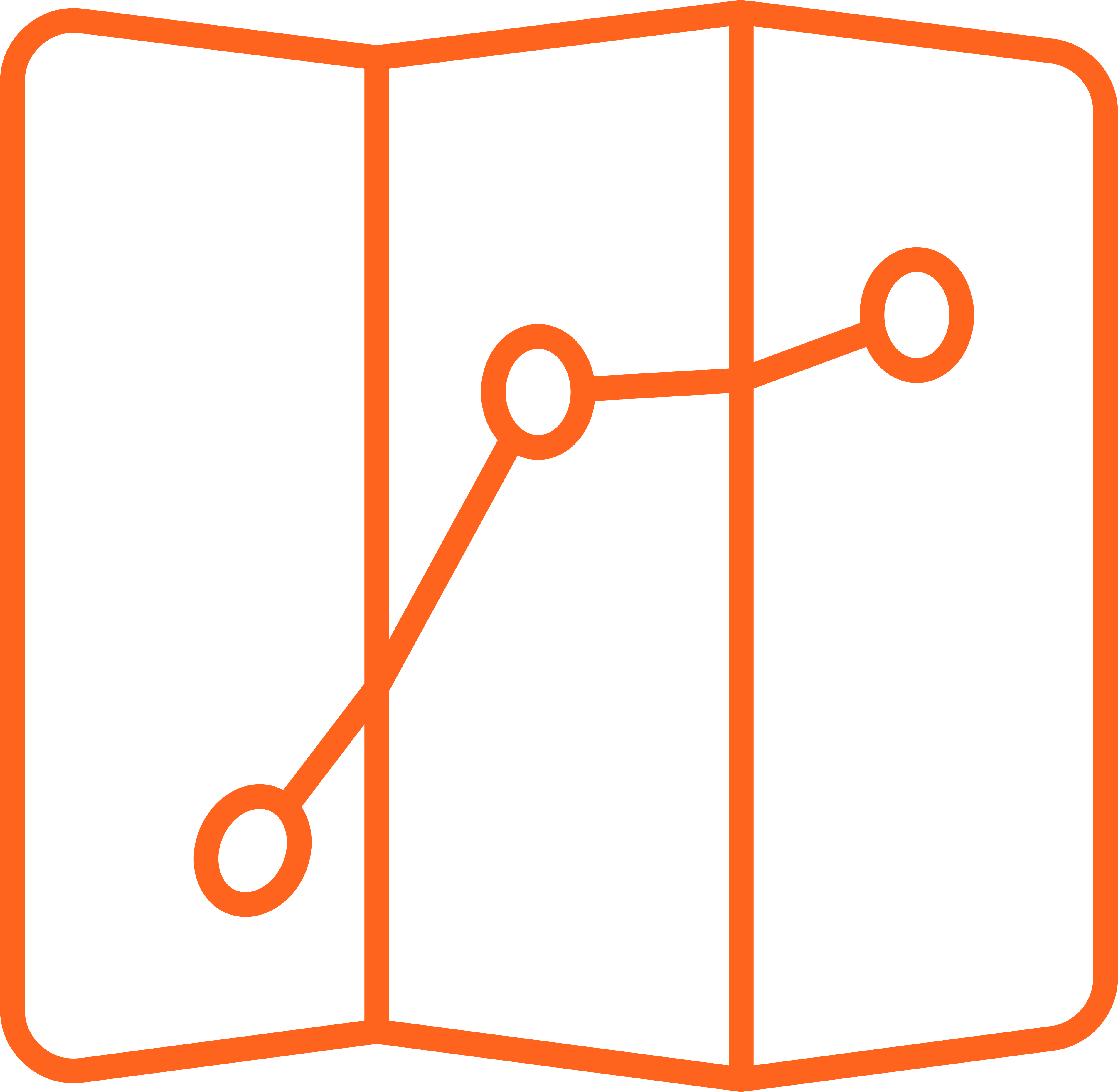
Oh dear.
On some journeys, the destination does matter.
We are sorry, we can't find that page
Please do report the problem so we can fix it.
This site has many pages and many visitors. We'd really appreciate your letting us know if there is something wrong.
If you are looking for a report and the link you are using contains “reports” in the URL, swap this out for “publications” and the link should work. E.g. If your link is trl.co.uk/reports/PPR212 then edit your link to trl.co.uk/publications/PPR212
Home